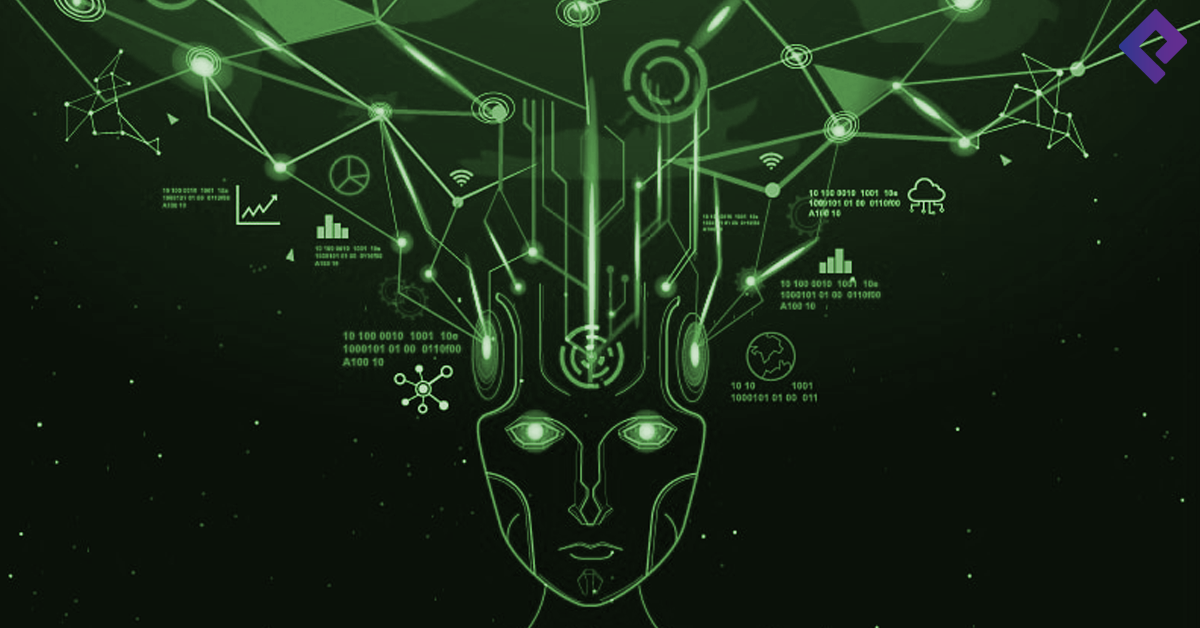
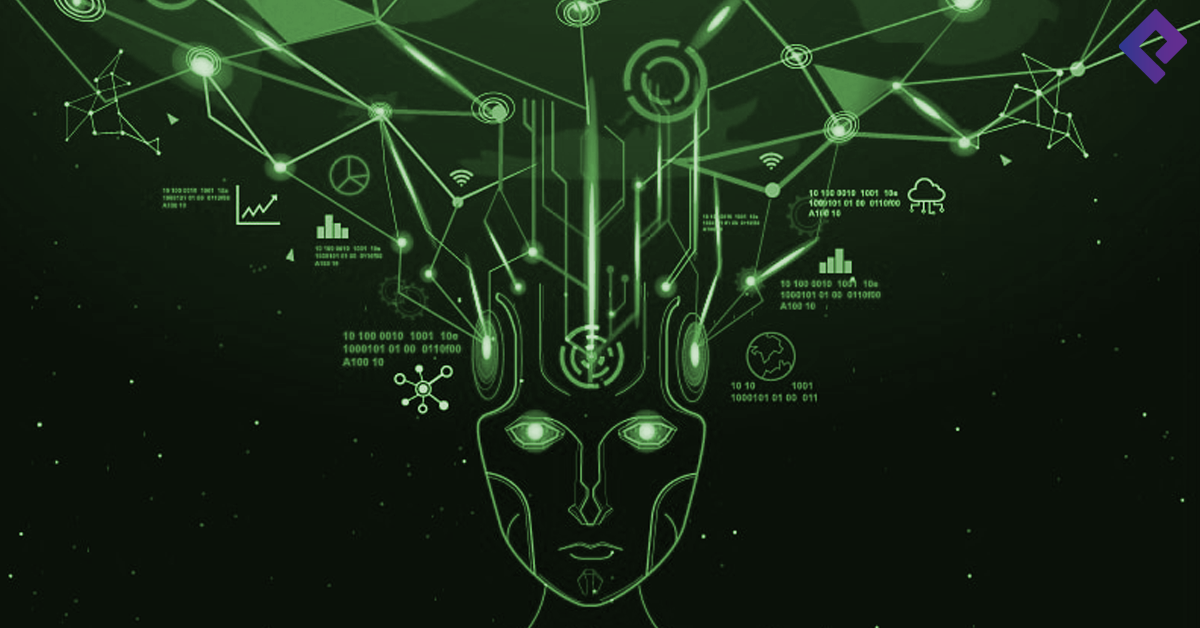
A large language model (LLM) is a specialized category of artificial intelligence (AI) and generative AI algorithms. LLMs use specific pre-programmed learning methods and an extensive amount of data sets to comprehend, summarize, produce and develop new content as it continues to learn. Below you can read more about the future of LLMs and how they are curated specifically for each company that implements a LLM.
The Future of Large Language Models
While LLMs offer a great deal of potential, it’s crucial to consider the ethical and societal implications that large language models may have, including potential biases in the training data and the environmental impact of training such large models. Therefore, the development and use of LLMs should be done responsibly and transparently.
Better Understanding of Human Language
Large language models can understand and generate human-like text. They are trained on vast amounts of data, enabling them to grasp the intricacies, context, and nuances of human language. This makes interactions with technology more natural and intuitive.
Numerous Practical Applications for Business
Large language models are used in a wide range of applications, from chatbots to content generation, translation services, and even voice assistants. They have also been used for more complex tasks like drafting emails, writing code, and answering detailed questions about documents.
Improving Access to Information
By understanding natural language queries, large language models can help people find and understand information more effectively. This is especially useful for those who may not know the exact keywords to search for or how to phrase their queries.
Bridging Communication Gaps
Large language models can translate languages, helping to break down barriers in communication. They can also be used to make complex or technical information more accessible to non-specialists.
Potential in Emerging Fields
There are potential applications in areas like mental health (e.g., providing initial assessments or therapeutic conversations), education (e.g., providing personalized learning experiences), and entertainment (e.g., creating stories or games).
How Large Language Models are Built
Building a large language model is a complex task that requires significant data and a large amount of business resources. However, when done correctly these LLMs can provide immense value to a company and streamline numerous processes.
Understanding the Problem
The first step involves identifying the problem that the language model will solve. This could range from responding to customer inquiries and generating content, to analyzing complex internal documents. The model’s purpose will inform the data it’s trained on and how it’s evaluated.
Data Collection and Preparation
Based on the identified use case, you would need to gather a large amount of text data that the model can learn from. In a company-specific context, this could include company documents, chat logs, emails, or other relevant text data. This data must then be cleaned and formatted appropriately for training the LLM.
Training the Large Language Model
The core of building a large language model is the training process. This involves inputing the prepared data into a machine learning algorithm (like a transformer-based model), which learns to predict the next word in a sentence based on the previous words or phrases. This training phase can take a significant amount of time and computational resources, depending on the size of the LLM and the amount of data.
Fine-Tuning
After the model has been trained on the general data, it’s often fine-tuned on more specific data that’s closer to the final tasks it will be performing. For instance, if the model is meant to answer customer service inquiries, it could be fine-tuned on a dataset of previous customer interactions from a chatbot or even customer emails.
Evaluation and Iteration
Once the model is trained and fine-tuned, it needs to be evaluated to ensure it meets the company’s needs and security measures. This evaluation phase might reveal areas where the LLM needs further tuning or even additional training data. The process of training, fine-tuning, and evaluation is crucial and may need to be repeated multiple times to achieve the desired performance.
Deployment and Monitoring
After the model has been trained and evaluated, it can be deployed into the company’s system if already implemented, or hosted on the providers platform. Once deployed, the model’s performance should be continually monitored and adjustments made as needed.
Ethical Considerations
Throughout this process, it’s vital to consider the ethical implications of the model. This includes ensuring the data used for training does not contain biases and that the model’s use complies with data privacy regulations such as PCI-DSS and HIPAA compliances.
Plum Voice Large Language Model Solutions
Secure LLMS – Automated Dialogs
The scalability and continued effectiveness of self-service dialog applications have reached unprecedented heights. Leveraging interactive voice response (IVR) and conversational AI, companies can interact in a way that is effective, quick to respond, provide ROI, and are in line with compliance security requirements. Plum Voice’s all-encompassing dialog solutions on an AI cloud platform operate non-stop with round-the-clock support, making us perfect for automating business operations. Our tools empower customers to conveniently place orders, process payments, access account information, schedule appointments, and more, all through their communication channels of choice.